25.05.2023
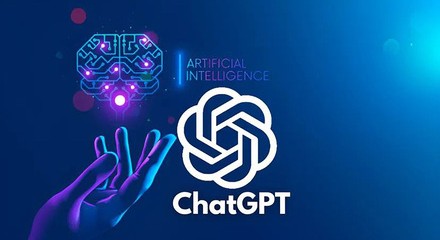
Patterns, prejudices and interests
It can be fun to discover the limits of chatbots, says Yassamine Mather, but democratic control is vital. AI will be used as a weapon in the class war
In recent weeks, the media has been full of alarming headlines about artificial intelligence.
For example, in The Guardian we have: “‘Godfather of AI’ Geoffrey Hinton quits Google and warns over dangers of misinformation” (he says the “dangers of chatbots were ‘quite scary’ and warns they could be exploited by ‘bad actors’”).1 Then there is The New York Times with “OpenAI’s Sam Altman urges AI regulation in Senate hearing”.2 He explains: “I think if this technology goes wrong, it can go quite wrong. And we want to be vocal about that … We want to work with the government to prevent that from happening.”
With the advent of and easy access to the ‘chatbot’ - a computer programme which imitates human conversation - many have reported obvious, at times dangerous, errors in this latest AI tool. One of the most talked about recent examples is that of a US law professor, wrongly accused of sexual harassment. Jonathan Turley claims he was falsely accused by the ChatGPT chatbot of assaulting students on a trip he “never took”, while working at a school he “never taught at”. Turley added: “What is most striking is that this false accusation was not just generated by AI but ostensibly based on a [Washington] Post article that never existed.”3
All this at a time when stories about prospects of mass unemployment caused by AI are also making headlines. According to the Financial Times, quoting Goldman Sachs,
The investment bank said on Monday that “generative” AI systems such as ChatGPT, which can create content that is indistinguishable from human output, could spark a productivity boom that would eventually raise annual global gross domestic product by seven percent over a 10-year period ... They calculate that roughly two-thirds of jobs in the US and Europe are exposed to some degree of AI automation, based on data on the tasks typically performed in thousands of occupations (May 23).
Of course, the defenders of AI will tell you the exact opposite. According to these people, AI improves efficiency, brings down costs and accelerates research - they say its development is the most influential invention ever, it will revolutionise human development and, when it comes to job losses, it will create many new jobs, so there is nothing to worry about.
The truth lies somewhere in between. My own investigation of ChatGPT has shown mixed results. Although replies to technical or mathematical questions are pretty accurate, those relating to more general information can be bizarre. Incredibly, the response to the same question can vary minute by minute - presumably as machine learning updates the system’s database.
In reply to my question about the academic journal Critique, ChatGPT gave reasonably accurate information about founder Hillel Ticktin, the journal’s origins and its analyses of the Soviet Union. It went on to say I had been the acting editor, but died in 2020! Yet this was described as a current journal, so I am not sure who is supposed to have acted as editor in the last 2.5 years. A question on the Middle East Centre in the University of Oxford gave completely false information, telling me that a well known professor of Iranian studies in the University of St Andrews was working at Oxford University.
Of course, this latest AI tool, learning from the more publicised mistakes of the last few weeks, is covering its back by adding the comment that you should visit/consult the relevant university/publisher ... page, as it will have more up-to-date information. ChatGPT is far more modest if you ask a medical question, telling me: “I’m not a doctor, but I can provide some general information that might be helpful”. It went on to inform me: “Ultimately, the decision to operate should be made after careful consideration of your individual health history, risk factors, and discussion with your healthcare provider.”
Limits
Contrary to all the hype, AI is obviously nowhere near being able to take over decision-making. It works best, not as capitalism wants us to believe, as a replacement of the human brain, but in conjunction with it. And the current danger is not simply from AI itself, but from state and other institutions that are willing to allow AI in its current infantile state - with all its mistakes, doubts and constant self-corrections - to create ‘definite’ final data sources, which can determine the plight of human beings. The disasters recorded so far have been the direct result of failing to check AI-generated data.
In the US, AI-based information on a Malaysian academic’s alleged membership of an Islamic group that was affiliated to a terrorist group led to a long court case. Rahinah Ibrahim, who had been a postgraduate in Stanford University, ended up on a US-based ‘Muslim no-fly’ list and her US visa was revoked. The US government has since been forced to accept that Ibrahim - currently the dean of architecture at University of Putra Malaysia - was never a national security threat. In her case false AI information, which was entered into a government database, led to her travel ban. Basically AI confused two similar-sounding, but very different, names of Islamic organisations - one associated with a professional Malaysian academic group that happened to have the word ‘Islam’ in it and the other an Islamist group. It is likely that if a human had checked the two names, they would have detected the very obvious difference - not something that an algorithm designed to identify spelling mistakes can do!
An integral and crucial part of artificial intelligence has been the development of ‘machine learning’ (ML) - often described as a subset of AI. However, ML in reality means feeding large amounts of data into algorithms, with the aim of teaching machines to recognise similar patterns, to learn from them and use this learning to make predictions. Obviously errors can occur in the gathered data - not to mention prejudices in terms of race, gender, accent, etc, with these faults becoming embedded in the algorithm. Yet the ML used in a wide range of daily applications - ranging from image and speech recognition to immigration controls and fraud detection - remains ‘supreme’ and is often unchallenged.
A simple example of ‘prejudice’ - in this case the influence of Eurocentrism - is provided by YouTube’s transcribing service, which converts recorded audio to plain text. It works reasonably well for North American or standard English accents, but you hit problems if the speaker has a strong regional accent and you might as well give up if they have an Indian or African accent. This is a direct consequence of the training material used by code writers who relied on audio files recorded in California. All other AI prejudices, including Islamophobia, have the same source. In other words, it is not simply AI, but the errors resulting from human input - which often remain unchallenged.
Another important AI development has been ‘deep learning’. This is more sophisticated, in that it makes use of neural networks - algorithms inspired by the more complicated structure of the human brain. Deep learning models can recognise complex patterns in pictures, text, sounds and other data to produce accurate insights and predictions, making them very useful for tasks like image and speech recognition.
However, some scientists have criticised reinforcement learning, which is a trial-and-error process. Basically an AI agent performs a number of actions in a given environment, and in each unique moment has a particular state, moving from one such state to a new one. The ‘software agent’ learns through trial and error, in that, when it takes a desired action, it receives a ‘reward’, in the same way as a pet is rewarded for good behaviour (or is punished for bad behaviour). In AI the rewards and punishments are calculated mathematically. For example, a self-driving system could receive a ‘-1’ when the model hits a wall, and a ‘+1’ if it safely passes another vehicle. These signals allow the agent to evaluate its performance.
The algorithm learns through trial and error to maximise the reward - and ultimately complete the task. This has been compared to what biological intelligence systems do. Therefore, we can say that each learning episode (sometimes called an ‘epoch’) can be represented as a sequence of states, actions and rewards. Each state depends only on the previous states and actions, as the environment is inherently stochastic (in other words, the state that follows next cannot be accurately predicted). The problem with all this is, of course, that trial and error is not very sophisticated - there is no room for pausing and reassessing the algorithm, the fundamental suppositions, etc.
There are plenty of examples of deep-learning AI going wrong. One such example was Amazon’s AI-based recruitment solution, which was supposed to be able to process résumés sent by job applicants, analyse their qualifications and other details, and provide a list of those who should be hired. However, it was soon discovered that the AI was ‘biased’ towards male candidates! Why was this? For the simple reason that Amazon’s engineers had bench-marked the neural network training data for an engineering job applicant’s résumé with the current (largely male) employees of engineering teams.
Employment
Capitalist use of AI has one fundamental aim: the maximisation of profit - often at the expense of reducing human involvement. We should, of course, be concerned about this - not just in terms of solidarity with those who will potentially lose their jobs, but also because this short-termism will inevitably lead to automation mistakes, if not disasters, and will ultimately have a damaging effect on the development of AI.
Let us look at the consequences of AI mistakes in some of the jobs known to be at risk. We are told, for instance, that AI will take on many of the tasks of a paralegal, including preparing legal documents, research, admin, providing quotes to clients, preparing questions for clients and witnesses, providing legal information … It will take just one case to go wrong for a legal firm to be taken to court, with subsequent litigation costing tens, if not hundreds, of thousands of pounds to stop this in its tracks.
Take the example of the driverless automated car. After billions of pounds were spent developing the AI for this to construct driving patterns and create databases, using data gathered by hundreds of thousands of Uber drivers, a couple of deaths caused by driverless cars in the US ended the practical deployment of such cars. I would hazard a guess that the first successful litigation against an AI-operated paralegal system would put an end to its use - despite all the current hype.
On the other hand, using AI to gather information, to categorise and analyse data in conjunction with a human paralegal, makes sense. Human beings can benefit from AI tools - as long as they remain in charge, rather than giving full control to mistake-prone AI decision-makers.
No doubt some jobs will be lost. We have recently seen pleas by supermarket checkout workers in the UK for shoppers not to use automated checkout points. Of course, all these automated points require regular human intervention, but one employee can oversee several checkpoints. Similarly, in airports the automated passport control, despite its many initial problems, seems to be working reasonably well, with face recognition software able to do the work previously carried out by a number of staff.
Supporters of AI claim it will actually create many new jobs. This may be true when it comes to developing new algorithms, but these jobs require highly trained staff and are currently mainly male. In the UK less than 10% of code writers are female. According to a statement on the United Nations website, UN Women, published in February 2023,
Today, women remain a minority in both STEM [science, technology, engineering and mathematics] education and careers, representing only 28% of engineering graduates, 22% of artificial intelligence workers and less than one third of tech-sector employees globally. Without equal representation in these fields, women’s participation in shaping technology, research, investments and policy will remain critically limited.4
Ironically the percentage is much lower in advanced western capitalist countries and, of course, this explains to certain extent the gender bias of many algorithms.
However, no-one seems to pay any attention to another bias in AI algorithms - that based on class!
-
www.theguardian.com/technology/2023/may/02/geoffrey-hinton-godfather-of-ai-quits-google-warns-dangers-of-machine-learning.↩︎
-
www.nytimes.com/2023/05/16/technology/openai-altman-artificial-intelligence-regulation.html.↩︎
-
eu.usatoday.com/story/opinion/columnist/2023/04/03/chatgpt-misinformation-bias-flaws-ai-chatbot/11571830002.↩︎
-
www.unwomen.org/en/news-stories/explainer/2023/02/power-on-how-we-can-supercharge-an-equitable-digital-future.↩︎